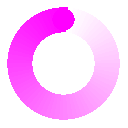
Master Weight of Evidence (WoE) & Information Value (IV) for Feature Selection | Predictive Modeling
📊 Understanding Weight of Evidence (WoE) & Information Value (IV) for Predictive Modeling
🔍 With Rajat | Data Science Simplified
In this video, Rajav breaks down two fundamental concepts in predictive modeling: Weight of Evidence (WoE) and Information Value (IV). These techniques are crucial for variable selection and are often featured in data science and analytics interviews.
📌 Timestamps & Highlights:
00:00 – Introduction to WoE & IV
00:27 – What is Weight of Evidence?
00:53 – Recap: WoE and its role in prediction
01:52 – Using the “default flag” as a dependent variable
02:31 – Binning salary ranges for WoE analysis
03:40 – Comparing good vs. bad customers by salary
04:22 – How to calculate WoE
04:56 – Interpreting example WoE values
05:38 – Transition to Information Value (IV)
06:12 – IV examples with salary & education
07:22 – Why WoE & IV matter in modeling and interviews
08:32 – Variable reduction using IV
09:05 – How to interpret IV scores
09:58 – IV score thresholds (0 to 1 scale)
12:21 – Calculating IV step-by-step
🧠 Key Takeaways:
WoE measures how well an independent variable predicts a target variable
IV quantifies the overall predictive strength of each feature
Learn how to bin variables, calculate WoE & IV, and use them for feature selection in modeling
Essential concepts for credit scoring, risk modeling, and ML preprocessing
👉 Don’t forget to like, subscribe, and hit the bell icon for more simplified data science tutorials.
#DataScience #MachineLearning #FeatureEngineering #WeightOfEvidence #InformationValue #PredictiveModeling #Analytics #rajatkumar #rajatExplains
コメント